Enlarge impact. Enhance sales. Spot talent. Get cited. Build communities. Read freely. Analyze complexities. These are just some of the promises made by the recent wave of software built to facilitate (digital) publishing. New media have always carried the promise of liberation and emancipation of (networks of) individual users from societal norms in ‘real life’, and publishing is not an exception. But are these promises viable? In this post, I will take a closer look at the politics of several exemplary applications developed to accommodate successful digital publishing with the use of automated (algorithmic) data-analysis.
Do these tools primarily serve the producers of publications, software developers, or marketeers? Will they allow writers and publishers to gain a proper insight in the current offer of publications in order to make it more diverse? Or do the metrics of their feedback loops result in echo chambers? Can these apps help to get the right publication to the right reader for the right reasons? Who even decides what is ‘right’ in these cases? Is software really able to differentiate between a lousy and a great text?
This is the first in a brief series of posts, each focusing on different steps in the production-consumption cycle of (digital) publishing. In this post, I discuss tools for marketing, post-publication management, and reading. In the follow-up post, I will write about peer-commenting and collaborative editing.
Marketing
A myriad of applications was developed to enhance marketing algorithmically. Some apps, like OptiQly, straightforwardly target sales. Others focus on different sorts of ‘impact’. Unsurprisingly, these apps have little concern for critical, academic or literary quality, instead focusing on quantifiable and marketable metrics. In the worst cases, they equal measurable marketability and quality.
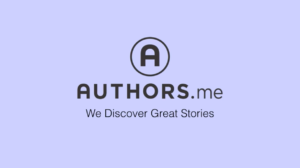
A good example of such algorithmic arrogance is Authors, an app which claims to ‘discover great stories’ by mere data-analysis of manuscripts. Authors is built to facilitate writers, scouts and publishers, feeding all parties advice based on the same algorithmic standards. I fear that the result of Authors’s approach is either a tautological and ultimately self-undermining feedback loop, or an equaling of demand and production. In any case, it nihilates the import of non-conformist creativity and makes one wonder: why not just let the algorithm write that great story itself?
Other marketing applications take a different route and combine algorithmic marketing with a social media approach. These for-profit applications like Mojo Reads function as platforms that generate a ‘community’ of readers and writers. They strive to achieve an infrastructural monopoly powered by a combination of game elements and sense of communality, no doubt having been inspired by Facebook.
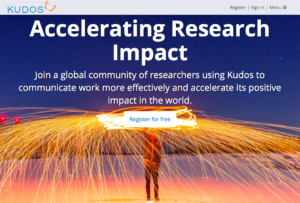
Interestingly, this social media approach is especially popular by applications with the aim to enhance the ‘impact’ of academic research publications like GrowKudos and LeanPub. This impact is not measured by sales, but by readership numbers and citations: a global and precarious peer-to-peer relationship which can be capitalized through social media logic.
Publication Management
The next step in the publishing cycle which uses data-analysis applications is post-publication management. The AltMetric bookmarklet can be used to track the citations of any text with a DOI, giving authors insight into their readership and effectivity. BuzzTrace offers the same service, with the additional features of managing social media content and protecting copyrights online. As such, these applications aim to serve and empower individual writers/publishers. Yet, it is striking how they attain to the traditional quantitative standards of academia and the market.
The measurement methods used might be innovative, but there is nothing alternative or emancipating to the type of data gathered and analyzed: citations, reads, purchases, downloads. Hence, providing individual authors with better insight into a publication’s impact in fact promotes better self-subjection to the market-dominated status quo of digital and academic publishing rather than individual agency. Imperative: Publish or Perish.
Reading
By contrast, some very different trends can be observed in the automated data-analysis software developed to assist readers. If publication management and marketing tools help to keep publications exclusive yet quantitatively successful, applications that use data-analysis to emancipate the reader make the entire publication-consumption cycle more inclusive. This should ultimately serve authors too.
A simple yet interesting example is Unpaywall. Using an extensive range of databases, the Unpaywall web extension traces free-of-charge versions of texts usually found behind paywalls. Circumventing paywalls thus is helpful for readers, but also beneficial for authors, for they reach broader audiences without losing any income themselves.
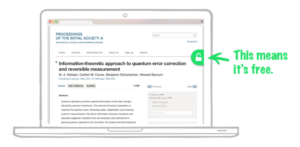
TopicGraph, a tool for automated textual analysis of contents of scholarly publications (PDFs), is an even richer example of emancipatory software development. TopicGraph highlights recurrent key terms and tracks them throughout a publication. Using scientifically developed linguistic analysis methods, it then combines these various key terms to create a page-to-page topic model. Such topic model does not only provide valuable insights to readers and researchers, it is also extremely helpful for writers to track the semantic structure of their own writings systematically. Being open-source and not-for-profit, this application is one of the few using automated text analysis to improve understanding and critical reflection rather than performance and impact. As such, it is one of the few yet precious examples of emancipatory software development in the realm of automated data analysis for publishing.
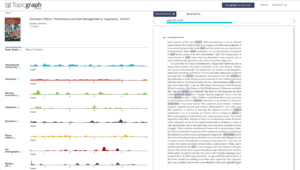
Conclusion (a non-algorithmic analysis)
All the software I discussed so far claims to make certain laborious tasks in the production-consumption cycle of publications easier. In a world with digital publishing possibilities that remain surprisingly poor, with high levels of precarity of academics, and with an unceasingly high threshold for high-quality publishing in general, these promises are tempting. It is clear, then, that the developers of these tools are on to something. But, as my colleagues noted in the Digital Publishing Toolkit, there is ‘a stark contrast between the fanciful promises of the computer industry and the often harsh reality of the new digital medium’. These applications might not be as helpful, as alternative, as innovative, and as emancipatory as they pretend to be.
All in all, it is clear that various trends in software development using automated data analysis signify different trends in the politics of (digital) publishing. Those tools focusing on marketing and post-publication management use ‘innovative’ technical means to uphold the status quo. With their metrics and feedback loops, they keep digital publishing uninventive, authors and researchers precarious, and they reinforce the power of the market and conservative academic (publishing) institutions. In the worst cases, the developers instrumentalize a fictitious sense of community, a feigned love of literature, and an unconvincing image of social entrepreneurship to sell their software.
One might be tempted to blame this algorithmic arrogance on the quantitative and homogenizing character of automated data-analysis itself. However, some open-source, non-profit applications prove otherwise by using automated data-analysis to make (the reading of) publications more sophisticated, critical, and inclusive. It shows that software can also be used for critical (and non-commercial) means.
My next post in this series on publishing tools in the digital age discusses collaborative editing and peer-help.